Blog
Discover Insights and Trends
Explore the latest insights in our articles on Data-centric AI, emphasizing the significance of quality data in AI models. Learn about innovative use cases for Industrial AI, including Retrieval-augmented generation (RAG).
Stay informed with our recent updates!
All posts
Read about our work and the latest news in the field of data-centric AI, industrial AI, LLMs, and more.
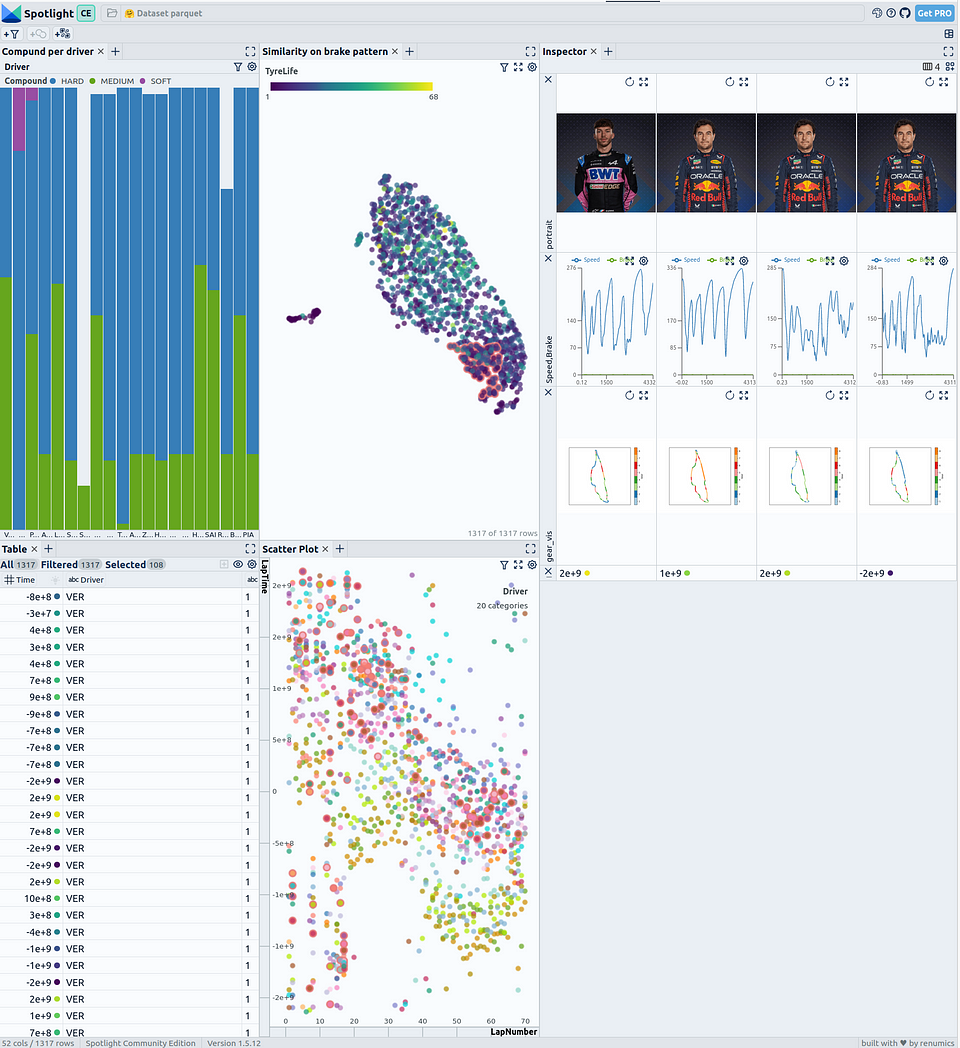
automotivetestingAI assistantsmachine learning
Automotive Testing: Automatic AI based Test Data Evaluation
An Example on Formula 1 Telemetry Data: Unsupervised AI for Time Series Preprocessing
Markus, Alexander, Stefan
June 18, 2024
•
6 min read
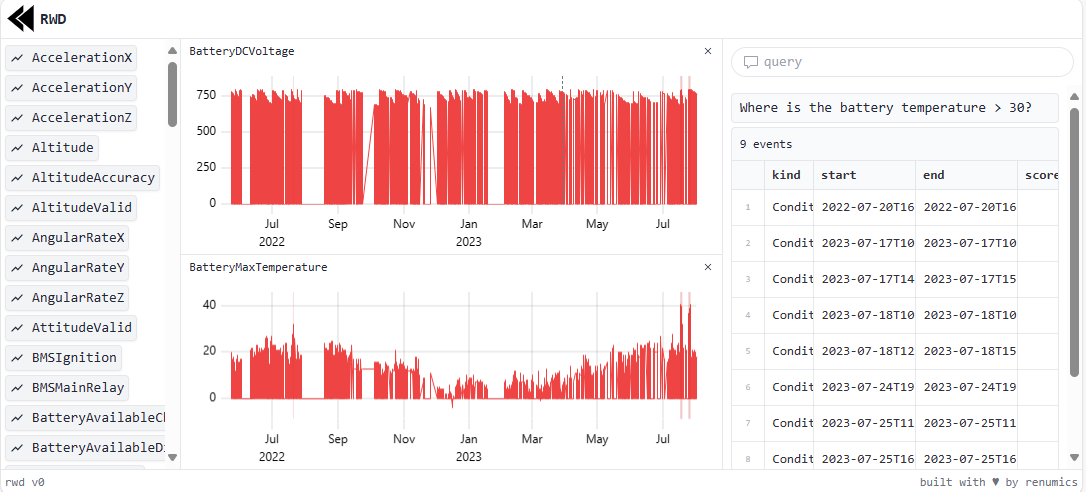
automotivetestingAI assistantsmachine learning
Top 5 Use Cases for AI in Automotive Testing Data Analysis
AI assistants can help test engineers to save valuable time. These 5 use cases illustrate how.
Stefan Suwelack, Markus Stoll
May 7, 2024
•
8 min read
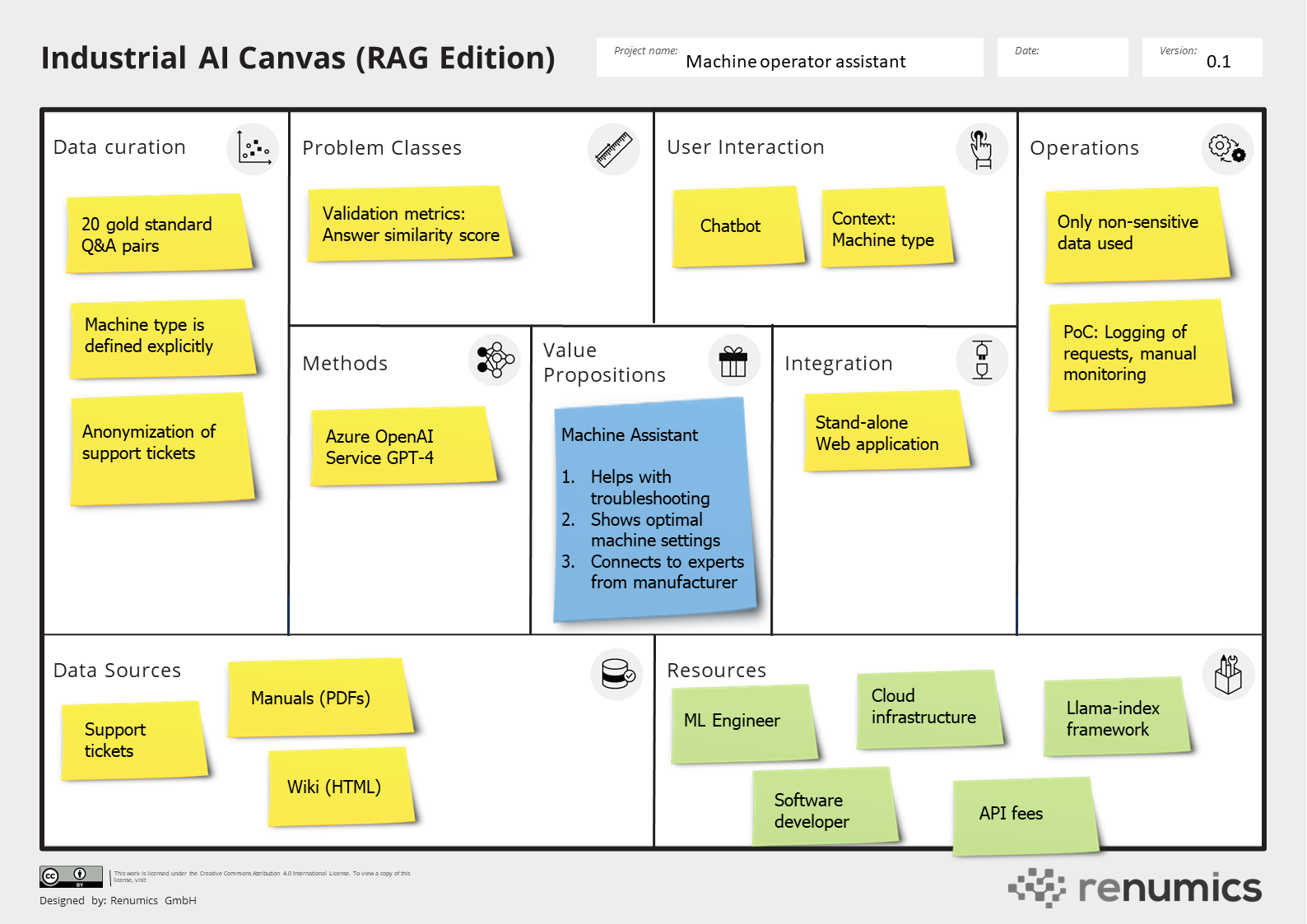
RAGdata-assistant
How to build RAG-based assistants for industrial applications
Retrieval augmented generation (RAG) can augment the knowledge of machine operators, allow for deeper insights into customer needs and enhance the collaboration of engineering teams. It has has emerged as the de-facto standard for building such applications-specific assistants.
Stefan Suwelack
December 19, 2023
•
7 min read
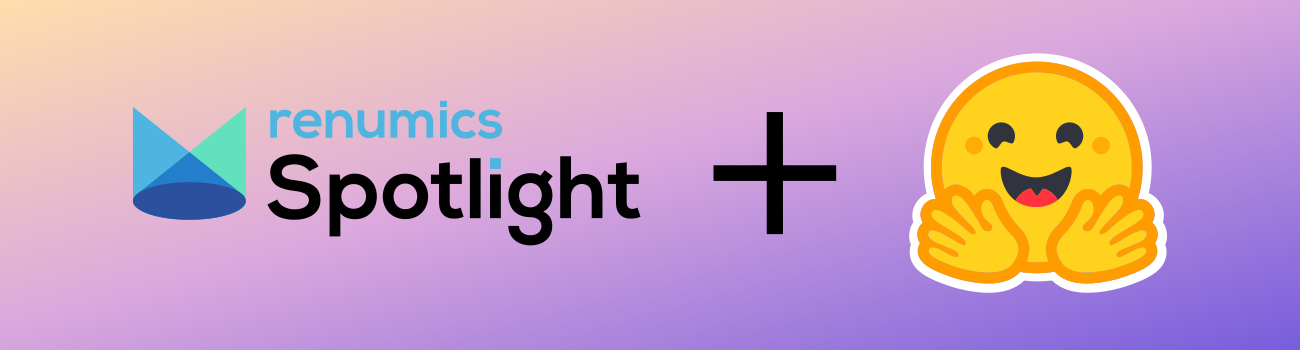
Interactive Visualization for Hugging Face Datasets
Hugging Face is the Github for AI. The platform helps you do discover new models and datasets. Now you can interactively inspect your Hugging Face datasets with just one line of code.
Stefan Suwelack
December 5, 2023
•
3 min read
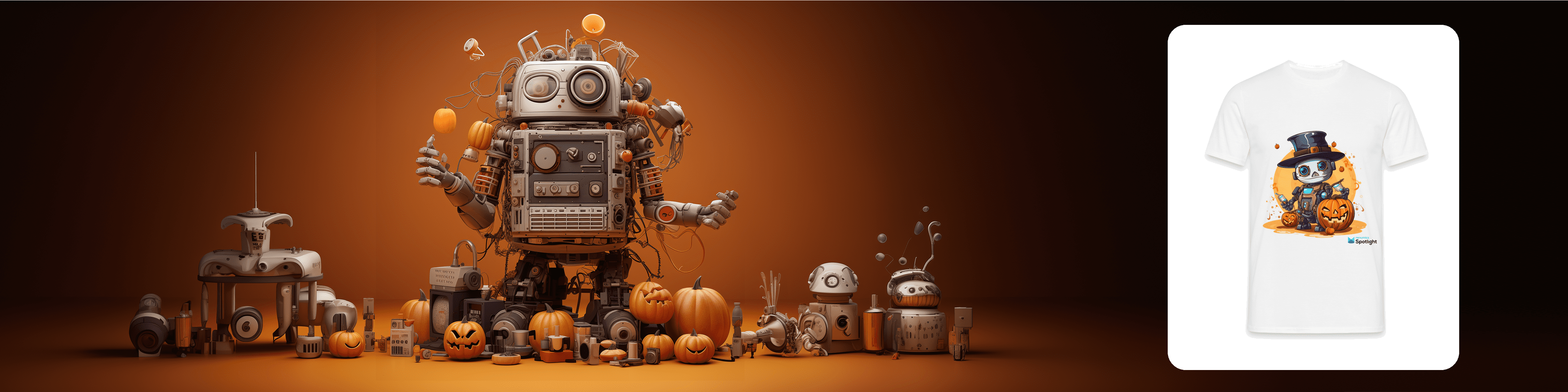
Join us for Hacktoberfest 2023
We’re thrilled to announce that Renumics Spotlight is joining the Hacktoberfest 2023. Every accepted Hacktoberfest PR not only elevates your skills and contributions to the open-source community but also earns you a limited-edition Renumics T-Shirt!
Markus Stoll
October 19, 2023
•
3 min read
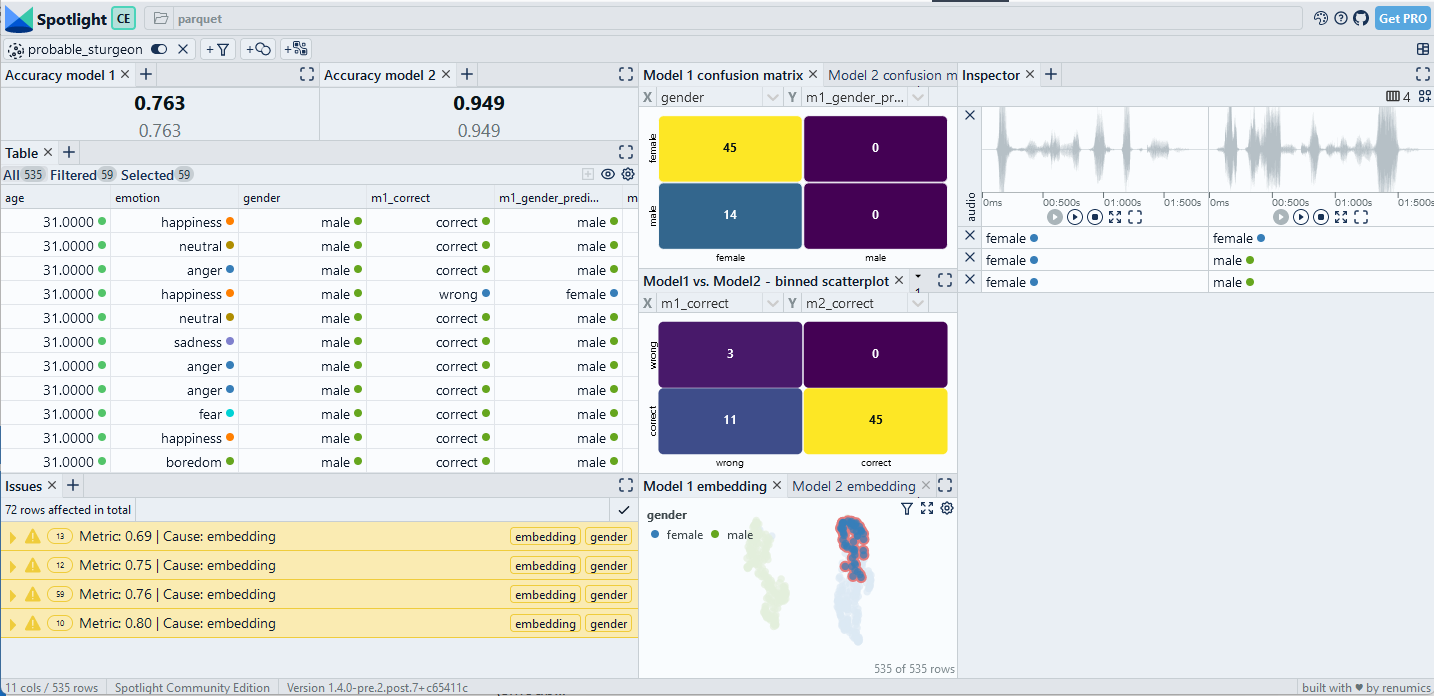
voice analyticsaudio mldata-centric ai
Hands-On Voice Analytics with Transformers
Voice analytics helps to build more sympathetic and more robust ML-based assistants. We show how to leverage open source tooling to effectively use pre-trained transformer models for this use case.
Stefan Suwelack
September 25, 2023
•
9 min read
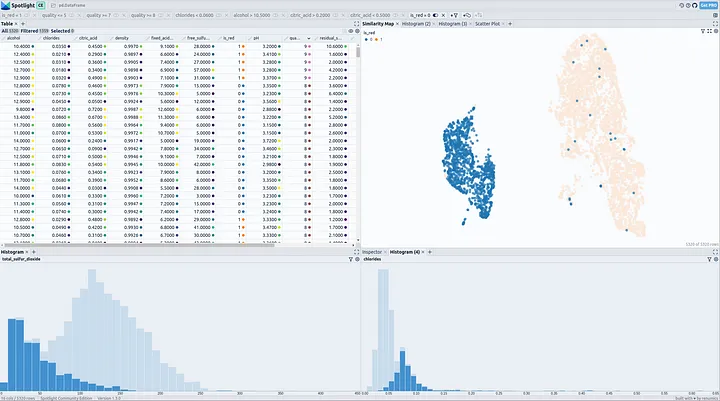
interactivedata visualizationdata explorationwine dataset
Interactive Data Insights Made Simple: Visualize with Just One Line of Code
Explore interactive data visualization with Spotlight. Dive into the wine dataset and uncover insights with our Open Source Tool.
Marius Steger
August 28, 2023
•
7 min read
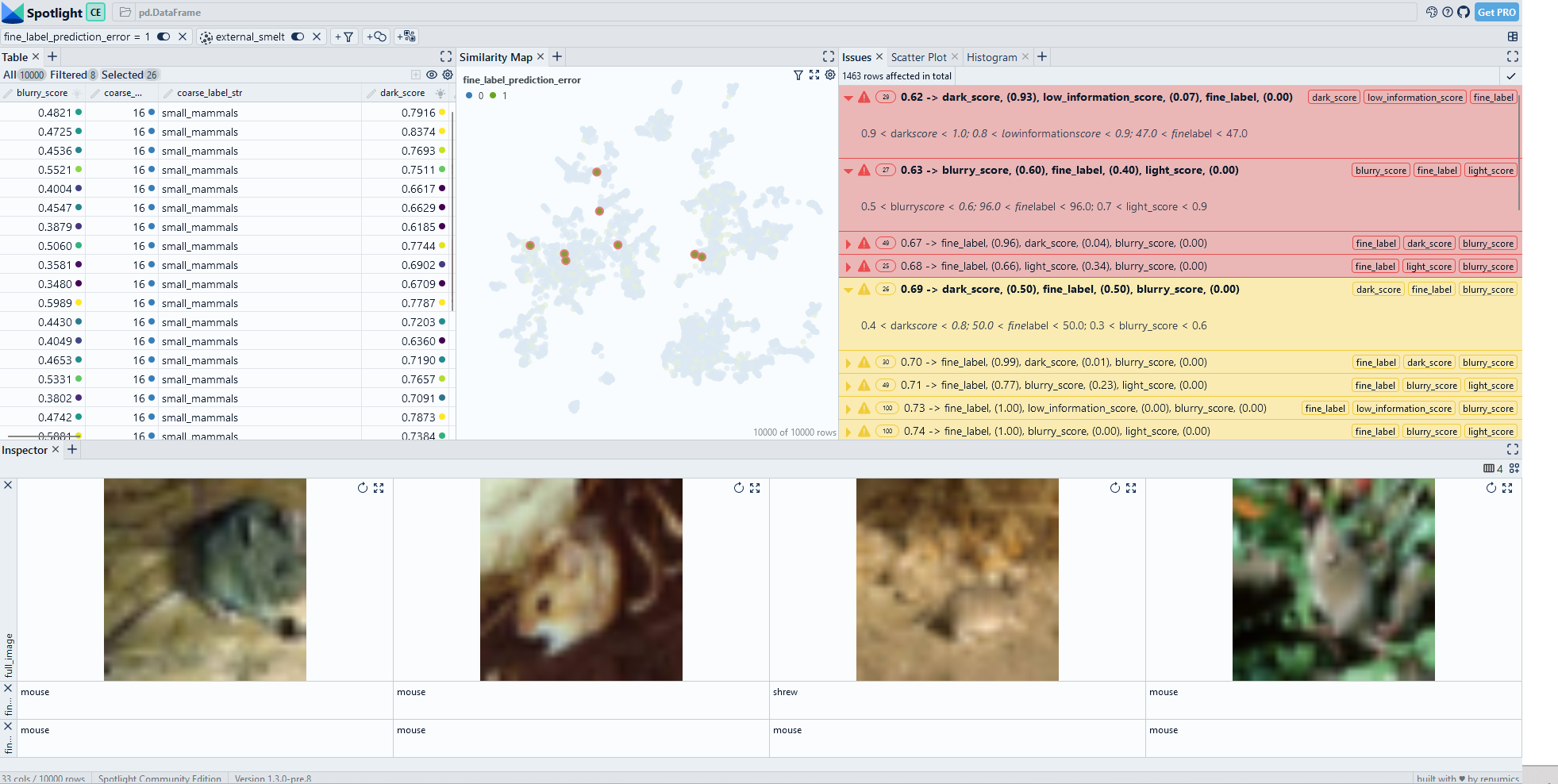
data-centric aidata slicingcomputer vision
Finding data slices in unstructured data
Data slices are semantically meaningful subsets of the data, where the model performs anomalously. We discuss current challenges and demonstrate hands-on examples of opens source tooling.
Stefan Suwelack
August 12, 2023
•
9 min read
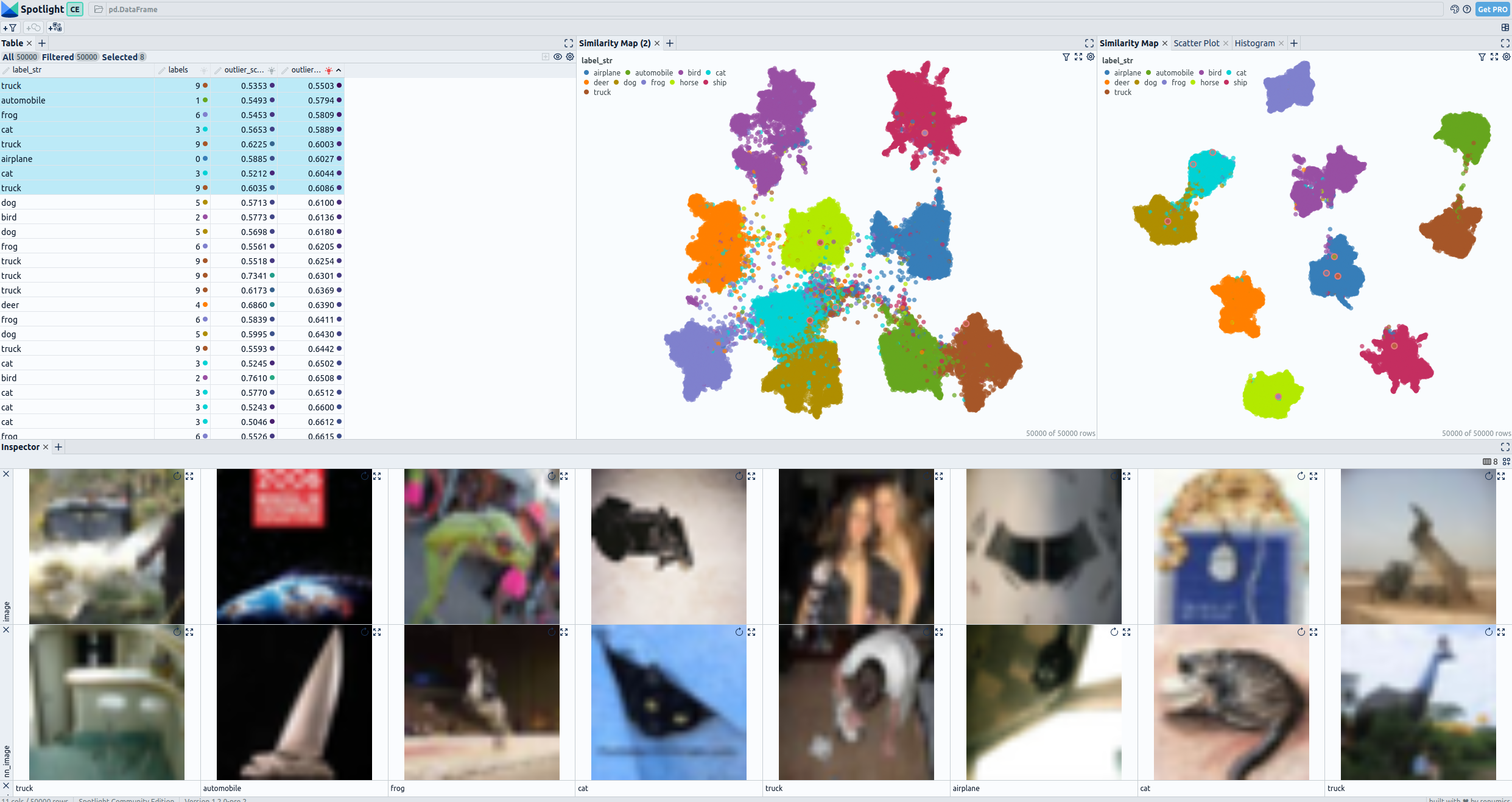
computer visiondata-visualizationdata-centric ai
Changes of Embeddings during Fine-Tuning of Vision Transformers (ViT)
Fine-tuning significantly influences embeddings in image classification. Pre-fine-tuning embeddings offer general-purpose representations, whereas post-fine-tuning embeddings capture task-specific features. This distinction can lead to varying outcomes in outlier detection and other tasks. Both pre-fine-tuning and post-fine-tuning embeddings have their unique strengths and should be used in combination to achieve a comprehensive analysis in image classification and analysis tasks.
Markus Stoll
July 27, 2023
•
10 min read
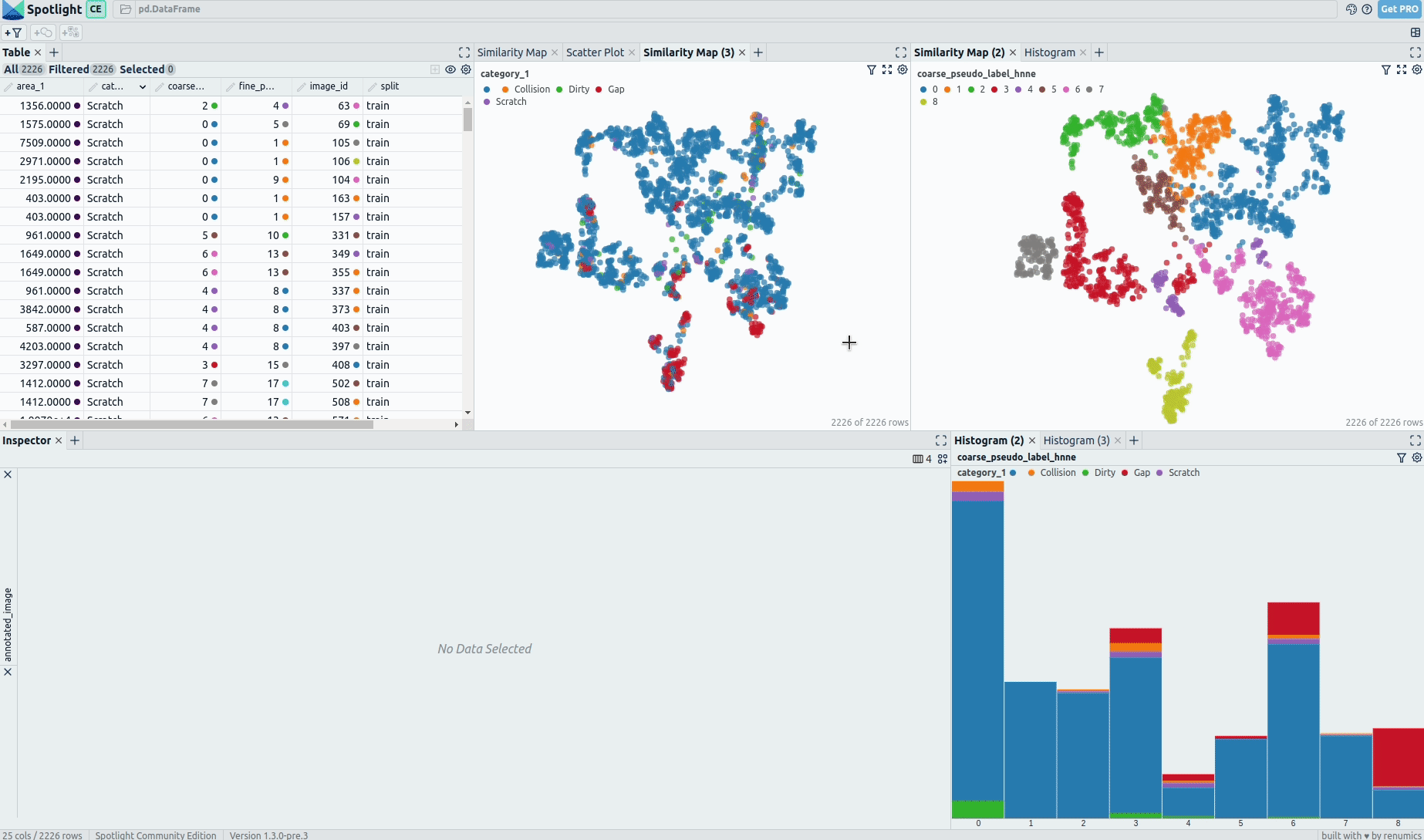
visualizationdata curationsynthetic data generationproductiondata-centric AIpythonclustering
Interactive Data Exploration with Spotlight: Unveiling Critical Segments to Guide Synthetic Data Generation
Building robust models for Visual Inspection in production settings can be a real challenge. Here, cloud services like Amazon Lookout for Vision promise relief for model training but have limitations regarding data curation. This article explores those potential shortcomings and shows how to improve over them to leverage these services to the fullest.
Marius Steger
July 13, 2023
•
10 min read